Gaussian-Gamma collaborative filtering: a hierarchical Bayesian model for recommender systems
Journal article
Luo, C., Zhang, B., Xiang, Y. and Qi, M. 2017. Gaussian-Gamma collaborative filtering: a hierarchical Bayesian model for recommender systems. Journal of Computer and System Sciences. https://doi.org/10.1016/j.jcss.2017.03.007
Authors | Luo, C., Zhang, B., Xiang, Y. and Qi, M. |
---|---|
Abstract | The traditional collaborative filtering (CF) suffers from two key challenges, namely, the normal assumption that it is not robust, and it is difficult to set in advance the penalty terms of the latent features. We therefore propose a hierarchical Bayesian model-based CF and the related inference algorithm. Specifically, we impose a Gaussian-Gamma prior on the ratings, and the latent features. We show the model is more robust, and the penalty terms can be adapted automatically in the inference. We use Gibbs sampler for the inference and provide a statistical explanation. We verify the performance using both synthetic and real datasets |
Year | 2017 |
Journal | Journal of Computer and System Sciences |
Publisher | Elsevier |
ISSN | 0022-0000 |
Digital Object Identifier (DOI) | https://doi.org/10.1016/j.jcss.2017.03.007 |
Publication dates | |
27 Apr 2017 | |
Publication process dates | |
Deposited | 16 Jan 2018 |
Accepted | 25 Mar 2017 |
Accepted author manuscript | |
Output status | Published |
https://repository.canterbury.ac.uk/item/887x8/gaussian-gamma-collaborative-filtering-a-hierarchical-bayesian-model-for-recommender-systems
Download files
143
total views231
total downloads3
views this month3
downloads this month
Export as
Related outputs
Default clearing and ex-ante contagion in financial systems with a two-layer network structure
Qi, M., Ding, Y., Chun, Y., Liu, W. and Liu, J. 2025. Default clearing and ex-ante contagion in financial systems with a two-layer network structure. Communications in Nonlinear Science and Numerical Simulation. 142 (1), p. 108515. https://doi.org/10.1016/j.cnsns.2024.108515Two-stage trading mechanism in enabling the design and optimization of flexible resources interaction in smart grid
Qi, M., Chen, Y., Li, H., Liu, Y., Wu, S. and Li, X. 2024. Two-stage trading mechanism in enabling the design and optimization of flexible resources interaction in smart grid. Computing and Informatics. 43 (6), p. 1483–1515. https://doi.org/10.31577/cai_2024_6_1483Corporate fraud detection based on improved BP neural network
Qi, M., Liu, W., Liu, M,, Yan, C. and Zhang, L. 2024. Corporate fraud detection based on improved BP neural network. Computing and Informatics. 43 (3), pp. 611–632-611–632. https://doi.org/10.31577/cai_2024_3_611Normalizing flow based uncertainty estimation for deep regression analysis
Qi, M., Zhang, B., Sui, W, Li, M. and Huang, Z. 2024. Normalizing flow based uncertainty estimation for deep regression analysis. Neurocomputing. 585 (6), p. 127645. https://doi.org/10.1016/j.neucom.2024.127645Focusing on the golden skills of effective communication and collaboration to further enhance graduate employability attributes
O'Leary, S., McGee, M., Qi, M., Millns, S. and Eyden, A. 2024. Focusing on the golden skills of effective communication and collaboration to further enhance graduate employability attributes. Graduate College Working Papers.Feed-forward ANN with Random Forest Technique for Identifying malicious Internet of Things network intrusion
Obarafor, Victor, Qi, Mandi and Zhang, Leishi 2024. Feed-forward ANN with Random Forest Technique for Identifying malicious Internet of Things network intrusion. in: 2024 2nd International Conference on Cyber Resilience (ICCR) IEEE.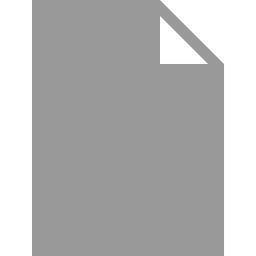
The role of use cases when adopting augmented reality into higher education pedagogy
Ward, G., Turner, S., Pitt, C., Qi, M., Richmond-Fuller, A. and Jackson, T. 2024. The role of use cases when adopting augmented reality into higher education pedagogy.A review of privacy-preserving federated learning, deep learning, and machine learning IIoT and IoTs solutions
Obarafor, Victor, Qi, Man and Zhang, L. 2023. A review of privacy-preserving federated learning, deep learning, and machine learning IIoT and IoTs solutions. in: 2023 8th IEEE International Conference on Signal and Image Processing (ICSIP) Wuxi, China IEEE. pp. 1074-1078Pre-processing of social media remarks for forensics
Gao, Xuhao and Qi, Man 2023. Pre-processing of social media remarks for forensics. IEEE. https://doi.org/10.1109/icnc-fskd59587.2023.10280980Alignment-based conformance checking of hierarchical process models
Wang, L, Han, X., Qi, M., Wang, K. and Lu, P. 2023. Alignment-based conformance checking of hierarchical process models. Computing and Informatics. 43 (1), pp. 149-180. https://doi.org/10.31577/cai_2024_1_149Research on UBI auto insurance pricing model based on parameter adaptive SAPSO optimal fuzzy controller
Wang, X., Liu, W., Ou, Z., Yan, C., Qi, M. and Jia, W. 2022. Research on UBI auto insurance pricing model based on parameter adaptive SAPSO optimal fuzzy controller. Computing and Informatics. 41 (4), pp. 1078-1113. https://doi.org/10.31577/cai_2022_4_1078Novel architecture for human re-identification with a two-stream neural network and attention ,echanism
Rahi, B. and Qi, M. 2022. Novel architecture for human re-identification with a two-stream neural network and attention ,echanism. Computing and Informatics. 41 (4), pp. 905-930. https://doi.org/10.31577/cai_2022_4_905A novel data analytic model for mining user insurance demands from microblogs
Chun Yan, Lu Liu, Wei Liu and Man Qi 2022. A novel data analytic model for mining user insurance demands from microblogs. Computing and Informatics. 41 (3). https://doi.org/10.31577/cai_2022_3_689Deep learning based real-time facial mask detection and crowd monitoring
Chan-Yun Yang, Hooman Samani, Nana Ji, Chunxu Li, Ding-Bang Chen and Man Qi 2022. Deep learning based real-time facial mask detection and crowd monitoring. Computing and Informatics. 40 (6), pp. 1263-11294. https://doi.org/10.31577/cai_2021_6_1263Repairing process models with non-free-choice constructs based on token replay
Bai, E., Qi, M., Luan, W., Li, P. and Du, Y. 2022. Repairing process models with non-free-choice constructs based on token replay. Computing and Informatics. 41 (4), pp. 1054-1077. https://doi.org/10.31577/cai_2022_4_1054Transformers only look once with nonlinear combination for real-time object detection
Xia, R., Li, G., Huang, Z., Pang, Y. and Qi, M. 2022. Transformers only look once with nonlinear combination for real-time object detection. Neural Computing and Applications. https://doi.org/10.1007/s00521-022-07333-yDeviation detection in clinical pathways based on business alignment
Tian, Y., Li, X., Qi, Man, Han, D. and Du, Yuyue 2022. Deviation detection in clinical pathways based on business alignment. Scientific Programming. 2022, pp. 1-13. https://doi.org/10.1155/2022/6993449Security vulnerabilities of popular smart home appliances
Qi, M., Induruwa, A. and Hussain, F. 2021. Security vulnerabilities of popular smart home appliances. in: Proceeding of The Twentieth International Conference on Networks April 18, 2021 to April 22, 2021 - Porto, PortugalImproved adaptive genetic algorithm for the vehicle insurance fraud identification model based on a BP neural network
Yan, C., Li, M., Liu, W. and Qi, M. 2020. Improved adaptive genetic algorithm for the vehicle insurance fraud identification model based on a BP neural network. Theoretical Computer Science. 817, pp. 12-23. https://doi.org/10.1016/j.tcs.2019.06.025Hybrid Intrusion Detection System for Smart Home Applications
Hussain, Fida, Induruwa, Abhaya and Qi, Man 2020. Hybrid Intrusion Detection System for Smart Home Applications. in: Mahmood, Z. (ed.) Developing and Monitoring Smart Environments for Intelligent Cities IGI Global. pp. 300-322Payments per claim model of outstanding claims reserve based on fuzzy linear regression
Yan, C., Liu, Q., Liu, J., Liu, W., Li, M. and Qi, M. 2019. Payments per claim model of outstanding claims reserve based on fuzzy linear regression. International Journal of Fuzzy Systems. 21, pp. 1950-1960. https://doi.org/10.1007/s40815-019-00617-xFuzzy interacting multiple model H∞ particle filter algorithm based on current statistical model
Wang, Q., Chen, X., Zhang, L., Li, J., Zhao, C. and Qi, M. 2019. Fuzzy interacting multiple model H∞ particle filter algorithm based on current statistical model. International Journal of Fuzzy Systems. 21, pp. 1894-1905. https://doi.org/10.1007/s40815-019-00678-yTemporal sparse feature auto-combination deep network for video action recognition
Wang, Q., Gong, D., Qi, M., Shen, Y. and Lei, Y. 2018. Temporal sparse feature auto-combination deep network for video action recognition. Concurrency and Computation: Practice and Experience. https://doi.org/10.1002/cpe.4487Soundness analytics of composed logical workflow nets
Liu, W., Wang, L., Feng, X., Qi, M., Yan, C. and Li, M. 2017. Soundness analytics of composed logical workflow nets. International Journal of Parallel Programming. https://doi.org/10.1007/s10766-017-0536-8A sliding window-based dynamic load balancing for heterogeneous Hadoop clusters
Liu, Y., Jing, W., Liu, Y., Lv, L., Qi, M. and Xiang, Y. 2016. A sliding window-based dynamic load balancing for heterogeneous Hadoop clusters. Concurrency and Computation: Practice and Experience. 29 (3). https://doi.org/10.1002/cpe.3763Facilitating visual surveillance with motion detections
Qi, M. 2017. Facilitating visual surveillance with motion detections. Concurrency and Computation: Practice and Experience. 29 (3). https://doi.org/10.1002/cpe.3770Data security of android applications
Obiri-Yeboah, J. and Qi, M. 2016. Data security of android applications. in: 2016 12th International Conference on Natural Computation, Fuzzy Systems and Knowledge Discovery : ICNC-FSKD 2016 : 13-15 August, Changsha, China IEEE Xplore.AL-DDCNN : a distributed crossing semantic gap learning for person re-identification
Cheng, K., Zhan, Y. and Qi, M. 2017. AL-DDCNN : a distributed crossing semantic gap learning for person re-identification. Concurrency and Computation: Practice and Experience. 29 (3). https://doi.org/10.1002/cpe.3766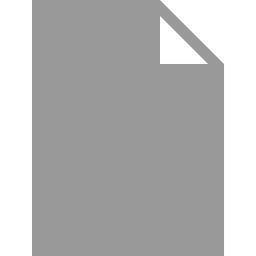