Evaluating the impact of artificial intelligence-assisted image analysis on the diagnostic accuracy of front-line clinicians in detecting fractures on plain X-rays (FRACT-AI): protocol for a prospective observational study.
Journal article
Novak, A., Hollowday, Max, Espinosa Morgado, A., Oke, Jason, Shelmerdine, S., Woznitza, N., Metcalfe, David, Costa, Matthew L, Wilson, S., Kiam, Jian Shen, Vaz, J., Limphaibool, N., Ventre, Jeanne, Jones, Daniel, Greenhalgh, Lois, Gleeson, Fergus, Welch, Nick, Mistry, Alpesh, Devic, Natasa, Teh, James and Ather, S. 2024. Evaluating the impact of artificial intelligence-assisted image analysis on the diagnostic accuracy of front-line clinicians in detecting fractures on plain X-rays (FRACT-AI): protocol for a prospective observational study. BMJ Open. 14 (9), p. e086061. https://doi.org/10.1136/bmjopen-2024-086061
Authors | Novak, A., Hollowday, Max, Espinosa Morgado, A., Oke, Jason, Shelmerdine, S., Woznitza, N., Metcalfe, David, Costa, Matthew L, Wilson, S., Kiam, Jian Shen, Vaz, J., Limphaibool, N., Ventre, Jeanne, Jones, Daniel, Greenhalgh, Lois, Gleeson, Fergus, Welch, Nick, Mistry, Alpesh, Devic, Natasa, Teh, James and Ather, S. |
---|---|
Abstract | Missed fractures are the most frequent diagnostic error attributed to clinicians in UK emergency departments and a significant cause of patient morbidity. Recently, advances in computer vision have led to artificial intelligence (AI)-enhanced model developments, which can support clinicians in the detection of fractures. Previous research has shown these models to have promising effects on diagnostic performance, but their impact on the diagnostic accuracy of clinicians in the National Health Service (NHS) setting has not yet been fully evaluated. A dataset of 500 plain radiographs derived from Oxford University Hospitals (OUH) NHS Foundation Trust will be collated to include all bones except the skull, facial bones and cervical spine. The dataset will be split evenly between radiographs showing one or more fractures and those without. The reference for each image will be established through independent review by two senior musculoskeletal radiologists. A third senior radiologist will resolve disagreements between two primary radiologists. The dataset will be analysed by a commercially available AI tool, BoneView (Gleamer, Paris, France), and its accuracy for detecting fractures will be determined with reference to the ground truth diagnosis. We will undertake a multiple case multiple reader study in which clinicians interpret all images without AI support, then repeat the process with access to AI algorithm output following a 4-week washout. 18 clinicians will be recruited as readers from four hospitals in England, from six distinct clinical groups, each with three levels of seniority (early-stage, mid-stage and later-stage career). Changes in the accuracy, confidence and speed of reporting will be compared with and without AI support. Readers will use a secure web-based DICOM (Digital Imaging and Communications in Medicine) viewer (www.raiqc.com), allowing radiograph viewing and abnormality identification. Pooled analyses will be reported for overall reader performance as well as for subgroups including clinical role, level of seniority, pathological finding and difficulty of image. The study has been approved by the UK Healthcare Research Authority (IRAS 310995, approved on 13 December 2022). The use of anonymised retrospective radiographs has been authorised by OUH NHS Foundation Trust. The results will be presented at relevant conferences and published in a peer-reviewed journal. This study is registered with ISRCTN (ISRCTN19562541) and ClinicalTrials.gov (NCT06130397). The paper reports the results of a substudy of STEDI2 (Simulation Training for Emergency Department Imaging Phase 2). [Abstract copyright: © Author(s) (or their employer(s)) 2024. Re-use permitted under CC BY. Published by BMJ.] |
Keywords | Diagnostic errors; Prospective studies; Fractures; Diagnostic imaging; Artificial intelligence; Research design; Radiography |
Year | 2024 |
Journal | BMJ Open |
Journal citation | 14 (9), p. e086061 |
Publisher | BMJ Publishing Group |
ISSN | 2044-6055 |
Digital Object Identifier (DOI) | https://doi.org/10.1136/bmjopen-2024-086061 |
Official URL | https://bmjopen.bmj.com/content/14/9/e086061 |
Publication dates | |
Online | 05 Sep 2024 |
Publication process dates | |
Accepted | 15 Aug 2024 |
Deposited | 17 Jan 2025 |
Publisher's version | License File Access Level Open |
Output status | Published |
Additional information | Publications router. |
Permalink -
https://repository.canterbury.ac.uk/item/99x4x/evaluating-the-impact-of-artificial-intelligence-assisted-image-analysis-on-the-diagnostic-accuracy-of-front-line-clinicians-in-detecting-fractures-on-plain-x-rays-fract-ai-protocol-for-a-prospective
Download files
23
total views9
total downloads2
views this month0
downloads this month
Export as
Related outputs
Data and data privacy impact assessments in the context of AI research and practice in the UK
Gilbert, Fiona J., Palmer, Jo, Woznitza, N., Nash, Jonathan, Brackstone, Carla, Faria, Lisa, Dunbar, J. Kevin, Hogg, Henry David Jeffry, Liu, Xiaoxuan and Denniston, Alastair K. 2025. Data and data privacy impact assessments in the context of AI research and practice in the UK. Frontiers in Health Services. 5, p. 1525955. https://doi.org/10.3389/frhs.2025.1525955Challenges for responsible AI design and workflow integration in healthcare: A case study of automatic feeding tube qualification in radiology
Thieme, A., Rajamohan, A., Cooper, B., Groombridge, H., Simister, R., Wong, Barney, Woznitza, N., Pinnock, M., Wetscherek, M., Morrison, C., Richardson, H., Pérez-García, F., Hyland, S., Bannur, S., Castro, D., Bouzid, K., Schwaighofer, A., Ranjit, M., Sharma, H., Lungren, M., Oktay, O., Alvarez-Valle, J., Nori, A., Harris, S. and Jacob, J. 2025. Challenges for responsible AI design and workflow integration in healthcare: A case study of automatic feeding tube qualification in radiology. ACM Transactions on Computer-Human Interaction. https://doi.org/10.1145/3716500An implementation facilitation intervention to improve the musculoskeletal X‑ray reporting by radiographers across London
Lockwood, P., Burton, C., Shaw, T., Woznitza, N., Compton, E., Groombridge, H., Hayes, N., Mane, U., O'Brien, A. and Patterson, S. 2025. An implementation facilitation intervention to improve the musculoskeletal X‑ray reporting by radiographers across London. BMC Health Services Research. 25 (248), p. 1. https://doi.org/10.1186/s12913-025-12356-xAccuracy of interpretation of nasogastric tube position on chest radiographs by diagnostic radiographers: A multi-case, multi-reader study
Creeden, A., McFadden, S., Ather, S. and Woznitza, N. 2025. Accuracy of interpretation of nasogastric tube position on chest radiographs by diagnostic radiographers: A multi-case, multi-reader study. Radiography. 31 (1), pp. 83-88. https://doi.org/10.1016/j.radi.2024.10.022Achieving earlier diagnosis of symptomatic lung cancer
Bradley, S., Baldwin, D., Bhartia, B., Black, G., Callister, Matthew Ej, Clayton, Karen, Eccles, Sinan R, Evison, Matthew, Fox, Jesme, Hamilton, W., Konya, J., Lee, Richard W, Bradley, S., Navani, Neal, Noble, Ben, Quaife, Samantha L, Randle, Amelia, Rawlinson, Janette, Richards, Michael, Woznitza, Nick and O'Dowd, Emma 2024. Achieving earlier diagnosis of symptomatic lung cancer. The British Journal of General Practice : The Journal of the Royal College of General Practitioners. 75 (750), pp. 40-43. https://doi.org/10.3399/bjgp25X740493Artificial intelligence (AI) for paediatric fracture detection: a multireader multicase (MRMC) study protocol.
Shelmerdine, S., Pauling, Cato, Allan, Emma, Langan, Dean, Ashworth, Emily, Yung, Ka-Wai, Barber, Joy, Haque, Saira, Rosewarne, David, Woznitza, N., Ather, S., Novak, A., Theivendran, Kanthan and Arthurs, O. 2024. Artificial intelligence (AI) for paediatric fracture detection: a multireader multicase (MRMC) study protocol. BMJ Open. 14 (12), p. e084448. https://doi.org/10.1136/bmjopen-2024-084448A survey of the NHS reporting radiographer workforce in England
Lockwood, P., Burton, C., Shaw, T. and Woznitza, N. 2024. A survey of the NHS reporting radiographer workforce in England. Radiography Open. 10 (1), pp. 1-18. https://doi.org/10.7577/radopen.5635Reporting radiographers within the European Federation of Radiographer Society (EFRS) member countries - motivation for becoming a reporting radiographer.
Jensen, J, Blackburn, P A, Gale, N, Senior, C, Woznitza, N, Heales, C J and Pedersen, M R V 2024. Reporting radiographers within the European Federation of Radiographer Society (EFRS) member countries - motivation for becoming a reporting radiographer. Radiography. 30 (3), pp. 731-736. https://doi.org/S1078-8174(24)00055-5AI assisted reader evaluation in acute CT head interpretation (AI-REACT): protocol for a multireader multicase study
Howell Fu, Alex Novak, Dennis Robert, Shamie Kumar, Swetha Tanamala, Jason Oke, Kanika Bhatia, Ruchir Shah, Andrea Romsauerova, Tilak Das, Abdalá Espinosa, Mariusz Tadeusz Grzeda, Mariapaola Narbone, Rahul Dharmadhikari, Mark Harrison, Kavitha Vimalesvaran, Jane Gooch, Nicholas Woznitza, Nabeeha Salik, Alan Campbell, Farhaan Khan, David J Lowe, Haris Shuaib and Sarim Ather 2024. AI assisted reader evaluation in acute CT head interpretation (AI-REACT): protocol for a multireader multicase study. BMJ Open. 14 (2), p. e079824. https://doi.org/10.1136/bmjopen-2023-079824Reporting radiographers in Europe survey: An overview of the role within the European Federation of Radiographer Society (EFRS) member countries
Pedersen, M.R. V., Jensen, J., Senior, C., Gale, N., Heales, C. J. and Woznitza, N. 2023. Reporting radiographers in Europe survey: An overview of the role within the European Federation of Radiographer Society (EFRS) member countries. Radiography. 29 (6), pp. 1100-1107. https://doi.org/10.1016/j.radi.2023.09.005Assessing the barriers and enablers to the implementation of the diagnostic radiographer musculoskeletal X‑ray reporting service within the NHS in England: a systematic literature review
Lockwood, P., Burton, C., Woznitza, N. and Shaw, T. 2023. Assessing the barriers and enablers to the implementation of the diagnostic radiographer musculoskeletal X‑ray reporting service within the NHS in England: a systematic literature review. BMC Health Services Research. 23 (1270), pp. 1-41. https://doi.org/10.1186/s12913-023-10161-y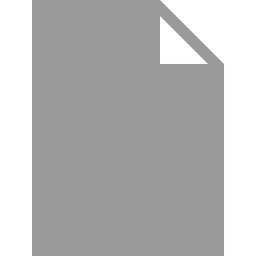