Dynamic decision support for resource offloading in heterogeneous Internet of Things environments
Journal article
Ali Jaddoa, Georgia Sakellari, Emmanouil Panaousis, George Loukas and Panagiotis G. Sarigiannidis 2020. Dynamic decision support for resource offloading in heterogeneous Internet of Things environments. Simulation Modelling Practice and Theory. 101. https://doi.org/10.1016/j.simpat.2019.102019
Authors | Ali Jaddoa, Georgia Sakellari, Emmanouil Panaousis, George Loukas and Panagiotis G. Sarigiannidis |
---|---|
Abstract | Computation offloading is one of the primary technological enablers of the Internet of Things (IoT), as it helps address individual devices’ resource restrictions. In the past, offloading would always utilise remote cloud infrastructures, but the increasing size of IoT data traffic and the real-time response requirements of modern and future IoT applications have led to the adoption of the edge computing paradigm, where the data is processed at the edge of the network. The decision as to whether cloud or edge resources will be utilised is typically taken at the design stage based on the type of the IoT device. Yet, the conditions that determine the optimality of this decision, such as the arrival rate, nature and sizes of the tasks, and crucially the real-time condition of the networks involved, keep changing. At the same time, the energy consumption of IoT devices is usually a key requirement, which is affected primarily by the time it takes to complete tasks, whether for the actual computation or for offloading them through the network. Here, we model the expected time and energy costs for the different options of offloading a task to the edge or the cloud, as well as of carrying out on the device itself. We use this model to allow the device to take the offloading decision dynamically as a new task arrives and based on the available information on the network connections and the states of the edge and the cloud. Having extended EdgeCloudSim to provide support for such dynamic decision making, we are able to compare this approach against IoT-first, edge-first, cloud-only, random and application-oriented probabilistic strategies. Our simulations on four different types of IoT applications show that allowing customisation and dynamic offloading decision support can improve drastically the response time of time-critical and small-size applications, and the energy consumption not only of the individual IoT devices but also of the system as a whole. This paves the way for future IoT devices that optimise their application response times, as well as their own energy autonomy and overall energy efficiency, in a decentralised and autonomous manner. |
Keywords | Internet of things; IoT offloading; Computation offloading; Edge computing; Decision support; Cloud computing |
Year | 2020 |
Journal | Simulation Modelling Practice and Theory |
Journal citation | 101 |
Publisher | Elsevier |
ISSN | 1569-190X |
Digital Object Identifier (DOI) | https://doi.org/10.1016/j.simpat.2019.102019 |
Official URL | https://www.sciencedirect.com/science/article/pii/S1569190X19301509 |
Publication dates | |
Online | 13 Nov 2019 |
Publication process dates | |
Accepted | 04 Nov 2019 |
Deposited | 27 Mar 2023 |
Output status | Published |
https://repository.canterbury.ac.uk/item/93q97/dynamic-decision-support-for-resource-offloading-in-heterogeneous-internet-of-things-environments
46
total views0
total downloads0
views this month0
downloads this month
Export as
Related outputs
A systematic review for the implication of generative AI in higher education
Al-Shabandar, R., Jaddoa, A., Elwi, T., Mohammed, A. and Hussain, A. 2024. A systematic review for the implication of generative AI in higher education. Infocommunications Journal. 16 (3), pp. 31-42. https://doi.org/10.36244/ICJ.2024.3.3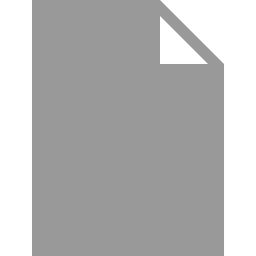